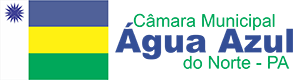
Atorlip-20
| Contato
Página Inicial

"Generic atorlip-20 20 mg overnight delivery, cholesterol test urine".
P. Tom, M.B. B.A.O., M.B.B.Ch., Ph.D.
Assistant Professor, Texas Tech University Health Sciences Center Paul L. Foster School of Medicine
Assessing statistical methods for coping with treatment switching in randomised managed trials: A simulation research cholesterol medication nightmares discount atorlip-20 20 mg visa. Estimating remedy effects from randomized clinical trials with noncompliance and loss to follow-up: the role of instrumental variable strategies cholesterol test results vldl atorlip-20 20 mg purchase free shipping. Every patient experiences these outcomes zinc cholesterol levels 20 mg atorlip-20 purchase with mastercard, although presumably after examine closure cholesterol levels lab values atorlip-20 20 mg order without a prescription, leading to censored information. Censoring is a serious purpose why these outcomes are analysed utilizing so-called survival evaluation. The log-rank test is the frequent check to compare therapy teams, and treatment results are sometimes quantified by means of a hazard ratio. If adjustment of the hazard ratio with respect to prognostic or predictive components is required, the Cox proportional hazards mannequin is often the regression strategy of selection. Use of these superior statistical techniques is properly established when learning efficacy, but the statistical evaluation of opposed occasions when studying safety usually is far more simplistic. This is each unusual and inadequate, as a result of the final knowledge structure, characterized by timing of events, censoring, and varying follow-up times, is similar for both efficacy and safety concerns. In many safety analyses, the two major workhorses to quantify the "threat" for a patient of experiencing at least one opposed event of a particular sort inside remedy groups are the incidence proportion and the incidence rate [2�8]. The incidence proportion is the variety of patients with an noticed opposed event divided by dimension of the group. Although widespread, 537 538 Textbook of Clinical Trials in Oncology the incidence proportion neither accounts for censoring nor for various follow-up occasions. The incidence fee has the identical numerator as the incidence proportion, however the denominator is the cumulative person-time at risk. The incidence fee is sometimes also referred to as exposureadjusted incidence price, if person-time at risk is simply counted whereas exposed. The incidence fee does account for both censoring and for various follow-up occasions. The ratio of the incidence rates between treatment groups is an estimator of a hazard ratio underneath the simplifying assumption that the hazards themselves, and not simply their ratio, are fixed over time. It is due to this simplifying assumption that use of the incidence fee is usually criticized. Perhaps more serious, nevertheless, is the restriction that the Kaplan�Meier-type approximation of the proportion of sufferers still alive and without an antagonistic events fails. In contrast, adverse occasions (of a specific type) could also be precluded by, for instance, demise. In statistical terminology, death with out prior opposed occasion known as a competing threat or a competing occasion. Time zero will often be time of first intake, probably after time of randomization, follow-up of adverse events could also be stopped after development of illness in oncological trials, time beneath subsequent therapies might (or might not) be included, follow-up may be targeted on opposed occasions of particular curiosity, and adverse occasions could additionally be distinguished between remedy emergent and never treatment emergent. We will nevertheless comment on censoring by disease progression (or some other illness event initiating subsequent therapy) in Section 25. It also permits us to spotlight the challenges imposed by censoring, which we contemplate in Section 25. A major cause is that, in our expertise, discussions then get shortly misplaced within the specifics of the security analysis at hand; see our feedback on our start line above. We have therefore chosen to simulate data inspired by the info example in Allignol et al. R code for simulation is offered in the online supplement in the companion web site of the book. Non-parametric analyses, accounting for competing dangers, are due to this fact mentioned in Section 25. Here, we discuss the frequent debate whether or not occurrence of antagonistic occasions is independently or informatively censored by. The security consequence of curiosity is time to first incidence of a selected opposed occasion. The opposed occasion may be recurrent, however we start with explaining the difficulties when analyzing time to first incidence. After progression, typically a second-line remedy is initiated and systematic follow-up of opposed occasions could also be stopped. In such a situation, progression is one other competing occasion, whereas the adverse occasion into account is "opposed occasion earlier than progression. Ci is the time to finish of follow-up without former statement of adverse or competing occasion. For clarity of presentation, we start with the whole information case with out censoring in Section 25. Recall, in the intervening time, that we assume complete follow-up, such that Ti is always smaller than Ci. The connection between the two measures of incidence is simple to see and it was already recognized to Florence Nightingale and William Farr of their nineteenth century cooperative work on opposed occasions in hospitals [10], but some later researchers appear to have lost sight of it. Rather, survival methodology [11] finds that the incidence rates estimate the event-specific hazards j (t) = lim P(T < t + t, = j t)/t, T t zero j = 1, 2, (25. When the event-specific hazards are time-varying, the formulas for survival operate and for the cumulative event probabilities might be more complex, however their kind will be comparable. Hence, computing ^ the incidence price 1 for adverse events without also computing the competing ^ incidence rate 2 is a somewhat incomplete analysis. In the absence of a competing event, the cumulative adverse event probability is given by 1 - exp (-t 1), (25. In the absence of censoring, the incidence proportion estimates the right-hand limit of the cumulative adverse occasion chance. With the usual limited follow-up and with the usual censoring, this will, normally, not be possible. Alternatively, it must depend on arguably questionable parametric extrapolation. This implies that the commonly used incidence proportion underestimates the "absolute risk of an anytime the Analysis of Adverse Events in Randomized Clinical Trials 543 n opposed occasion. This can be the reason ^ ^ ^ why one should be cautious, to say the least, about using 1/(1 + 2) for estimating P(= 1). The reason is that, in our experience, discussions then quickly digress, addressing questions corresponding to whether the adverse event into account is therapy associated or not. We have therefore chosen to simulate "opposed event" information following a constant eventspecific hazards mannequin, with and without censoring. For the time being, this allows us to shelve the question whether incidence charges are acceptable estimators and give attention to the following: � Demonstrate that cumulative antagonistic event chances will be overestimated if the competing-risks structure is ignored. Interpreting sort 2 events as "dying without prior opposed event," this selection implies that eventually-with "infinite" follow-up-two-thirds of all patients first expertise an antagonistic occasion and one-third of all patients die without prior antagonistic occasion. The curve is seen to strategy 100 percent, because it ignores the competing risks construction as explained earlier. This is a transparent case of overestimation, as a outcome of the true cumulative opposed occasion probability is bounded from above by two-thirds. In truth, assuming fixed event-specific hazards, incidence charges are constant estimators of the cumulative antagonistic occasion possibilities (Equation 25. However, censoring does disturb validity of the incidence proportion (Equation 25. Solid traces: the higher curve (dark grey) overestimates, the decrease curve (black) is the empirical counterpart of Equation 25. Of course, non- and semi-parametric methods of survival analysis additionally apply to learning adverse occasions. One complication is that they must account for competing dangers, however this is, after all, also true for parametric methods as seen earlier. That is, this the time to adverse event (= 1) or competing event (= 2), whatever happens first. In the straightforward setting of fixed event-specific hazards, we found that P(= 1) = 1 1 + 2 and P(= 2) = 2, 1 + 2 which also signifies that each patient experiences the composite event sooner or later in time (although possibly after closure of the study) as a result of P(= 1) + P(= 2) = 1. This is necessary as a end result of 1 - the Kaplan�Meier estimator then estimates the likelihood of experiencing the composite occasion, which tends to one with infinite follow-up. In such a situation, the estimated likelihood of experiencing the composite event must not are likely to one with ever longer follow-up as a result of there also is a optimistic chance to expertise the additional competing occasions.
The approaches offered on this chapter can work with a lot of candidate biomarkers without prior understanding of whether they have predictive power cholesterol lowering foods nhs 20 mg atorlip-20 cheap with amex. Statistical issues in the validation of prognostic cholesterol khan academy 20 mg atorlip-20 purchase free shipping, predictive cholesterol ratio desirable atorlip-20 20 mg purchase with mastercard, and surrogate biomarkers cholesterol in shrimp hdl or ldl trusted atorlip-20 20 mg. Guidance for industry: Enrichment methods for medical trials to support approval of human medication and organic merchandise. Tutorial in biostatistics: Data-driven subgroup identification and analysis in scientific trials. General steerage on exploratory and confirmatory subgroup evaluation in late-stage scientific trials. Methods for identification and affirmation of focused subgroups in scientific trials: A systematic review. Bayesian analysis of heterogeneous treatment effects for patient-centered outcomes research. An method to evaluating and comparing biomarkers for affected person treatment selection. Statistical Methods for Biomarker and Subgroup Evaluation in Oncology Trials 345 12. Identification of predicted individual treatment results in randomized medical trials. A regression tree method to figuring out subgroups with differential treatment effects. Regularized consequence weighted subgroup identification for differential remedy effects. Qualitative interaction bushes: A device to identify qualitative treatment-subgroup interactions. Bayesian two-step lasso strategy for biomarker choice in personalized drugs development for time-to-event endpoints. Estimating optimal therapy regimes via subgroup identification in randomized management trials and observational studies. Biomedicine and Clinical Trials Design, Chen Z, Liu A, Qu Y, Tang L, Ting N, Tsong Y, eds. Identification of subgroups with differential remedy effects for longitudinal and multiresponse variables. Using cross validation to evaluate the predictive accuracy of survival threat classifiers based on high dimensional knowledge. Subgroup analyses in randomised controlled trials: Quantifying the dangers of false-positives and false-negatives. Subgroup analysis in randomized controlled trials: Importance, indications, and interpretation. Prognostic components in oncology associate host and tumor variables to scientific outcomes independent of treatment [2]. On the other hand, predictive components are dependent on the treatment and are described by the interplay between the remedy and the consider predicting outcomes [2]. Lastly, environmental factors are ones which are exterior to the affected person, similar to entry to healthcare or to a cancer-control program [3]. Prognostic components 347 348 Textbook of Clinical Trials in Oncology play a significant function as they influence clinical outcomes, and the American Joint Committee on Cancer has established three criteria that outline a prognostic issue [3]. There are several the purpose why the identification of prognostic elements is important [4]. Understanding the advanced relationship between host and tumor-related factors and its impact on clinical outcomes is critical in order that (1) clinicians use the prognostic factors in the assessment of stage of illness, (2) investigators gain insights into the illness course of, (3) patients and their households are knowledgeable about prognosis, (4) enrichment methods could be developed to evaluate novel therapies and (5) medical trialists employ these components in the design and evaluation of clinical trials. This article covers matters related to both the design and analysis of prognostic factors, specializing in elements which would possibly be related on the time of prognosis or initial remedy. We concentrate on prognostic models, that are created by including a number of prognostic factors. The chapter first presents examples of how prognostic components are applied in the design and analysis of clinical trials after which discusses the design of prognostic components studies. The focus then shifts to sample dimension computation and an exploration of the completely different shrinkage strategies of variable selection. Next, common problems which would possibly be encountered in modeling prognostic factors are highlighted, followed by a succinct evaluate discussion of some nice advantages of the inner validation approaches and strategies for assessing prognostic models. The chapter concludes by endorsing the established criteria set by the American Joint Committee on Cancer [5], the Transparent Reporting of a Multivariate Prediction Model for Individual Prognosis or Diagnosis [6] and the Critical Appraisal and Data Extraction for Systematic Reviews of Prediction Modelling [7], in growing and validating prognostic fashions of clinical outcomes. As indicated above, prognostic elements may be the standard medical and pathologic factors, however they may also be genetic and molecular markers from the tumor. The prognostic elements may be mixed to create prognostic models that predict scientific outcomes. In sure situations it could be advantageous to use prognostic models within the randomization versus a number of prognostic elements. Randomization was stratified by the anticipated survival likelihood determined by a prognostic model of overall survival [8]. The main goal of utilizing a prognostic model in A031201 is to steadiness the important baseline components by the therapy task. Developing and Validating Prognostic Models of Clinical Outcomes 349 Risk models have been also employed for screening eligible patients on recent trials. High-risk men had been recognized by the Kattan nomogram if their predicted probability of being illness free 5 years after surgical procedure <60% [11]. The OncotypeDx is a 21-gene rating that predicts likelihood of recurrence and has been extensively validated [15]. Women with intermediate threat of recurrence (that is an OncotypeDx risk rating 11�25) have been randomized to endocrine therapy or endocrine therapy plus chemotherapy. The major goal was testing whether or not intermediate-risk girls randomized to endocrine remedy plus chemotherapy have non-inferior disease-free survival to ladies handled with endocrine therapy alone. Prognostic components have been also used in enrichment trial design with a targeted therapy; these designs are also referred to as targeted designs. Historically, the motivation for the identification of prognostic elements is to estimate the effect of therapy accurately. Therefore, one other important utility of prognostic elements is adjusting prognostic elements so as to decrease bias in estimating the therapy impact. It is all the time recommended that the statistical test for the first analysis is pre-specified within the statistical evaluation plan. Thestratified log-rank check is the optimal test for estimating the treatment effect if the randomization is blocked on stratification elements. The major evaluation for the co-primary endpoints general survival and progression-free survival were based mostly on the stratified log-rank check. The stratified hazard ratio for general survival and progression-free survival had been zero. It is highly beneficial that investigators excited about identifying prognostic elements design the study prospectively. In different phrases, they need to observe the scientific research paradigm by stating a main speculation, defining the first end result a priori, and justifying the pattern size. Importantly, the sample dimension should be large enough due to the considerable number of potential biases that may occur when conducting such analyses. These points embrace lacking data of the variables or outcomes, variable-selection methods, multiple 350 Textbook of Clinical Trials in Oncology comparisons, and assessment of various models [18]. The reader is referred to Simon and Altman, who provide a rigorous review of statistical aspects of prognostic components studies in oncology [20]. Five hundred forty-nine sufferers with obtainable specimens consented that their plasma be used on this evaluation. If the correlation between the prognostic variables is factored in, then one would use the pattern measurement formulation developed by Schmoor et al. There is an ad-hoc rule of thumb where 15 events per variable are required for time-to-event endpoints; and 10 patients per variable are needed for a binary endpoint [4,23]. A better approach is to compute the standard error for the concordance index (c-index) [24]. We demonstrate how we carried out simulations to estimate the c-index and the variance of the c-index for the primary goal of growing a prognostic model that can predict total survival in men with metastatic castrate-resistant prostate most cancers who failed first line chemotherapy [25]. The objective was that once the final mannequin was chosen, the prediction error might be estimated on the model new dataset of patients [27]. Briefly, a proportional hazards relationship was generated from the Weibull regression model ti = exp(xi) * i the place is a vector of the regression coefficients for the covariates, xi is a vector for the covariates for each subject i. The noticed survival time is the minimum between failure time and the censoring time.
Often cholesterol test types buy generic atorlip-20 20 mg on-line, the total population is stratified into a biomarker-positive (B+) population (the sufferers having a sure genetic mutation or tumor development because of cholesterol food shrimp order 20 mg atorlip-20 free shipping the activity of a sure gene or pathway cholesterol heart disease atorlip-20 20 mg order, for example) and a biomarker-negative (B-) inhabitants (all different patients) cholesterol lowering supplement order atorlip-20 20 mg fast delivery. Sometimes, the dichotomization into B+ and B- can be subject to misclassification error. In each cases (investigation of multiple endpoint or investigation of multiple subpopulation), applicable statistical strategies are wanted to take care of the fact that simultaneous a number of inference is done on a research question. As one other reflection of the bias, assume that a degree estimate and a confidence interval for the treatment impact are calculated separately in each of a quantity of subpopulations, based mostly solely on data from that subpopulation. Subsequently, solely the most important level estimate and its confidence interval are reported. This is misleading, because the treatment effect estimate will be overestimated and the arrogance interval "shifted upward" because of the choice of the reported outcome. We have mentioned the two most common sources of multiplicity in oncology trials, however other sources are also relevant at instances, for instance, � Multiple therapy arms, usually both completely different doses or totally different dosing regimens for a treatment, � Simultaneous comparisons of an experimental drug with an active comparator and a placebo group, � Combination drug therapies. Another common supply of multiplicity is the repeated inspection of accruing information in group-sequential and adaptive trials. From the perspective of statistical methodology, the approaches used for this share many similarities with multiple testing procedures used for the multiplicity conditions just described. Specifically, in both fields, the joint distribution of check statistics are sometimes assumed to be multivariate regular with some correlation construction [2]. The difference lies in the fact that in group-sequential approaches, a number of particular person hypotheses are tested repeatedly with different quantities of information out there. Group-sequential methods are the topic of Chapters 9 and 12 and thus not mentioned right here intimately. Testing for important differences between therapy choices is a key method in confirmatory clinical trials and has been the primary target of a lot analysis into multiplicity-adjustment methodology. We subsequently continue with a review of a quantity of testing procedures together with their graphical illustration [6]. We conclude with some strategic and operational considerations for multiplicity adjustments in oncology. For example, consider a new compound being investigated for efficacy claims in three disjoint and unbiased populations, with each hypothesis tested at level 0. The probability of falsely claiming that the compound has a positive treatment impact in no much less than one of the three populations is 1 -(1 -0. Before conducting the trial, we do not know whether or not the compound is efficacious in all or some populations, or in another setting, for all or some endpoints. On the opposite hand, the weak sense solely considers the configuration during which all null hypotheses are true. Another kind of error fee is becoming extra incessantly thought of, particularly when exploring many hypotheses. The false discovery fee is the anticipated proportion of falsely rejected hypotheses among the rejected hypotheses. An example of a stepwise procedure is the Holm [10] procedure, which can additionally be a stepwise extension of the Bonferroni check. For tumor response rate, �1 is the imply distinction between the responder charges of the remedy and the management group. All seven intersection hypotheses could be tested utilizing an -level take a look at such as the Bonferroni test mentioned in Section 10. For instance, the elementary hypothesis H1 is rejected if and provided that H1 H 2 H three, H1 H 2, H1 H 3 and H1 are all rejected. Alternatively, one also can regulate p-values so that they are often compared with the general significance stage. Formally, the adjusted p-value is outlined because the smallest significance stage at which a hypothesis could be rejected by the chosen a number of testing process [14]. As an instance, the Bonferroni test for three hypotheses has the adjusted critical value of /3. Hence, if the p-values for the three hypotheses are p1, p2, and p3, then the Bonferroni take a look at rejects Hi if pi /3, i = 1, 2, three. Alternatively, the decision procedure can be described by way of the adjusted p-values 3p1, 3p2, 3p3, which suggests Hi is rejected if 3pi, i = 1, 2, three. Loosely speaking, for single-step procedures, the acceptance area of the a number of check defines a multiple confidence area for the treatment-effect estimates. Further discussions are supplied in Brannath and Schmidt [15] and Strassburger and Bretz [16]. In its easiest version, it splits the total significance degree equally among m null hypotheses and checks every null speculation at degree /m. From another angle, we can view the m hypotheses as being weighted equally with weights 1/m. In apply, nonetheless, some hypotheses could also be more related than others because of scientific importance, likelihood of rejecting, and so on. To handle this, weighted versions of the Bonferroni m test assign completely different weights wi to hypotheses Hi, the place i=1 wi = 1. A speculation is rejected by the weighted Bonferroni test if its p-value is lower than or equal to wi. However, they have an inclination to be fairly conservative when the check statistics are extremely correlated. The procedure first orders the p-values from the smallest to the biggest: p(1) p(2) � � � p(m). If H(1) is rejected, the process exams H(2) and rejects it if p(2) /(m - 1); in any other case, testing stops. If H(2) can be rejected, the procedure checks H(3) and rejects it if p(3) /(m - 2); otherwise, testing stops. It may be shown that the Holm procedure is a closed testing procedure which applies the Bonferroni take a look at to every intersection speculation. It can be shown that the Hochberg process rejects all hypotheses rejected by the Holm process, and probably extra [17]. For example, if the joint distribution is a multivariate normal distribution, the situation requires that the correlations should be non-negative. These considerations lead to a number of testing strategies which take such considerations under consideration. One simple but common gatekeeping structure is that key secondary hypotheses are tested provided that the first speculation has been rejected. In this case, the primary hypothesis is the "gatekeeper" for key secondary hypotheses. Many gatekeeping procedures in addition to other complex multiple testing strategies could be visualized in a graphical procedure [6]. Another easy example is the fixed-sequence (also known as strictly hierarchical) check [20]. A fastened order of hypotheses is determined before data are collected, then testing proceeds sequentially in this order. Here, w1 = 1 in the node H1 indicates that H1 is initially examined at degree 1 �, whereas H2 is examined initially at degree zero �. If H1 can be rejected, 1 � is shifted to H2, as indicated by the sting weight g12 = 1 on top of the sting from H1 to H2. If any inequality is happy, the corresponding hypothesis (H1 or H2) can also be rejected and 1 1 2 is propagated to the opposite hypothesis which is then tested at degree (1/2) + (1/2) =. The preliminary weights fulfill i wi 1 and the sting weights also fulfill j gij 1 for all nodes i, the place g ij is the edge weight associated with the edge from Hi to Hj. Multiple Comparisons, Multiple Primary Endpoints and Subpopulation Analysis 191 the potential combinations of endpoints and populations, and correspondingly many attainable multiplicity-adjustment strategies have been mentioned. For illustrative functions, we now talk about one of many thought of methods, though finally not carried out within the actual research. If this requirement is met, additional hypotheses may be tested at possibly greater significance levels. The levels wi �, and the weights gij on the sides are updated based on the algorithm given by Bretz et al.
This includes remedy measured as a binary variable cholesterol levels germany buy 20 mg atorlip-20 overnight delivery, modification of some 427 428 Textbook of Clinical Trials in Oncology physiological parameters measured as continuous variables fasting cholesterol definition atorlip-20 20 mg amex, or time-to-event outcomes cholesterol belongs to which class of molecules 20 mg atorlip-20 order fast delivery. Those final endpoints are thought of because the gold commonplace however they can be tough to assess if they require invasive procedures or in depth exams; they can also be long to acquire cholesterol free definition atorlip-20 20 mg generic overnight delivery, in particular for time-to-event outcomes, or be so rare that the statistical energy is inadequate to detect meaningful clinical impact sizes with typical pattern sizes. Examples embrace analysis of cardiovascular events in hypertension illness [1], despair scores, time to dying after resectable colon cancer [2], or death in localized prostate cancer [3]. For instance, blood strain could be a surrogate of cardiovascular events; disease-free survival and metastasis-free survival may be surrogates of overall survival in colon and prostate most cancers, respectively. Not solely can surrogate endpoints potentially handle the above-mentioned limits of ultimate endpoints, but they can be also mixed to improve the quantity of data provided by a medical trial [4]. For occasion, in oncology, overall survival is classically thought-about because the gold normal to consider remedies; nonetheless, in case of accessible efficacy outcomes on an intermediate endpoint, such as disease progression, physicians could select the most effective therapy primarily based on what has been administered or could suggest the investigational remedies to sufferers who were in the control arm leading to biases in the evaluation of the final endpoints. Actually, in the literature, two totally different viewpoints are typically confronted: the first one provides the preeminence to the organic or physiological rationale within the evaluation of the validity of surrogate endpoints. The surrogate endpoint must be based on a key mechanism that determines the worth of the final endpoint: modifying the surrogate will result in a modification of the ultimate endpoint. Stuart Baker, in a latest article [8], lists 5 criteria for utilizing surrogate endpoints, amongst which three of them contain "scientific and biological considerations: similarity of organic mechanisms of treatments between the brand new trial and previous trials, similarity of secondary treatments following the surrogate endpoint between the model new trial and previous trials, and a negligible danger of harmful side effects arising after the remark of the surrogate endpoint in the new trial. This offers method to the excellence between the individual and the trial-level surrogacy, a central notion in this subject. The case where each the surrogate and the ultimate endpoints are steady variables serves to present some of the models and ideas. Next, the precise case of each outcomes being time-to-event endpoints is explored. Finally, we touch upon the robustness of the findings to validate surrogate endpoints. We use examples in early breast cancer and in abdomen most cancers that we briefly introduce within the following section. Advanced/recurrent ailments have a very poor prognosis as most sufferers ultimately die before 2 years. There is then a strong want for higher treatments and accelerated medical trials. This known as the trial-level surrogacy, which can additionally be expressed as the power to predict the effect of remedy on the ultimate endpoint from the impact of therapy on the intermediate endpoint. As was reminded more than 30 years in the past [16], the analysis of the connection between response to therapy and time to occasion may be biased if the response to therapy is measured after initiation of follow-up. The starting date for the time-to-event endpoint is postponed in order that the response is measured earlier than the initiation of the follow-up. Censored observations and occasions occurring before the landmark time of response analysis are removed from the evaluation. The surrogate and last endpoints are correlated, the remedy impact on the surrogate is non-null, the therapy impact on the final endpoint is non-null, and the treatment impact on the final endpoint disappears after adjustment on the surrogate endpoint. In addition to the patient-level correlation (condition 1), Prentice launched the notion of the surrogate being on the "pathway" between the intervention (the treatment) and the ultimate endpoint. Operational criteria have then been derived by Freedman, however he also highlighted three limitations, making those theoretical criteria troublesome to apply in apply [19]. The capability to detect a remedy effect (reject or not the null hypothesis) instantly is dependent upon the pattern dimension. A measure of the "extent" to which an endpoint meets the Prentice criteria seems extra helpful in follow. He proposed to evaluate the decrease confidence restrict of this proportion to some threshold. This situation can solely be verified if the remedy has a massively important effect on the final endpoint, a rare state of affairs. This falls within the framework of measuring information achieve and explained randomness. This can be interpreted as a proportion of variation of the ultimate endpoint defined by the surrogate endpoint. Two units of knowledge with completely different amounts of censored remark, however having the identical distribution of events and leading to the identical estimates of therapy impact, might not give the same defined variation. This limit is frequent to most measures of defined variation applied on censored knowledge. This can be a difficulty within the context of the analysis of surrogate endpoints evaluated on several trials with totally different follow-up. He proposed utilizing the information gain,, that measures the space between two fashions, one indexed by the parameter related to the remedy indicator Z, the other listed by the worth zero. The transformation 2(Z) = 1 - exp- can be seen as the proportion of randomness defined by the regression. The authors provide a quantity of estimators which are impartial of the censoring mechanism a minimum of when censoring is unbiased of the event course of. Building on the causal inference framework, a quantity of approaches have been proposed. To introduce some notation, let us assume that a new therapy (Z = 2) is compared to a control (Z = 1). Let us assume that in a digital world, every patient might be treated with each therapies. The two potential surrogate and true outcomes for affected person j are famous S2j, S1j, T2j, T1j, the place the primary subscript denotes the therapy. One can then outline the remedy effect at the particular person stage: as (Tj) and (Sj). Of course, in the true world, only Assessing the Value of Surrogate Endpoints 433 the outcomes S and T within the allocated arm are observable and the s are non-measurable, nor can they be estimated except untestable assumptions are made. Various publications differ primarily by the sort of assumptions postulated to estimate these portions. It is possible to remedy the non-identifiability drawback by parameter restriction or the introduction of sensitivity parameters into the relationship between surrogate and ultimate endpoints. The latter authors confirmed the limitations of the individual causal impact because of the required untestable (and usually unrealistic) hypotheses. They proposed an alternative strategy counting on the expected causal effects somewhat than on the individual causal effect. Expected causal effect is solely the usual anticipated impact of treatment versus the control. Expected causal impact can then be expressed when it comes to previously developed measures because the relative impact [22] or explained variations. Unlike the individual causal effects, expected causal effects are estimable from the data under pretty general circumstances. Furthermore, one might argue that in a clinical trial context, anticipated causal results are central. In reality, regulatory agencies largely consider anticipated causal effects for granting commercialization licenses, and surrogate endpoints are primarily used as a tool to velocity up this means of approval. Each circle corresponds to a trial, the diameter of which is proportional to the sample size. In this example of stomach cancer, there seems to be a strong association between the 2 measures of treatment effect. This equation permits the researches to predict the remedy impact on the final endpoint from the treatment impact on the surrogate. The linear regression additionally provides us with a easy measure of trial-level association between the two endpoints: the coefficient of dedication, i. Finally, this linear regression also supplies 95% prediction limits across the treatment impact on T, given the estimated effect on S. The authors confirmed the shortage of affiliation on the trial level between treatment results measured by both endpoints. We will start with the best case of two continuous outcomes usually distributed, for ease of the presentation, after which describe the case of two time-to-event outcomes. As before, Tij and Sij are random variables denoting the true and surrogate endpoints for the jth topic in the ith trial, respectively, and let Zij be the indicator variable for treatment. One then has two easy linear fashions relating the remedy impact on the surrogate and on the true endpoint: Sij = �Si + iZij + Sij Tij = �Ti + iZij + Tij 436 Textbook of Clinical Trials in Oncology the place intercepts and remedy effects are trial-specific. The residual errors Sij and Tij, assumed to be usually distributed, could additionally be correlated as measured in the same patients/ trials. In different phrases, every estimated value �Si and i are random attracts from a distribution around the global �S and.
Atorlip-20 20 mg buy mastercard. MRSA - cholesterol inhibitor Naked Scientists podcast.